QuantaCell, IBM & CHU Jean Perrin team wins the JFR Challenge 2019 of the French Radiology Society
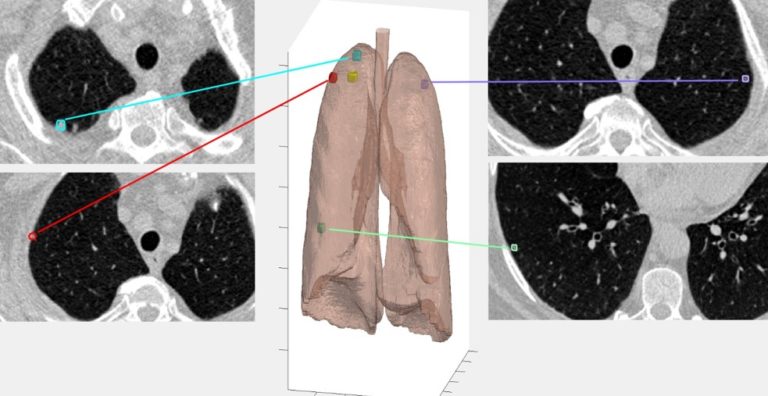
QuantaCell is proud to be part of the lung cancer nodule detection challenge with Dr Julien Brehant from University Hospital Jean Perrin (Clermont-Ferrand) and IBM Montpellier (Cognitive Systems).
https://community.ibm.com/community/user/ai-datascience/blogs/marc-fiammante1/2019/10/21/an-ibm-quantacell-team-wins-jfr-challenge
The objective of the challenge was to “distinguish 3D scanner exams with lung cancer nodules greater than 100mm3”
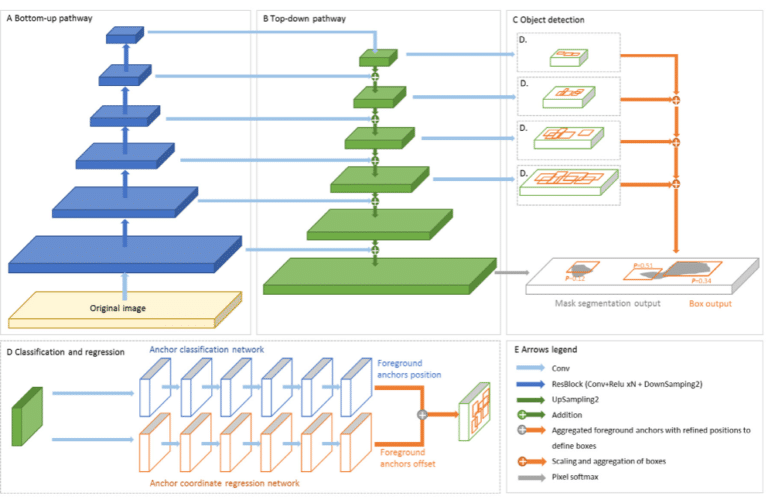
This challenge was a difficult detection problem because of the respiratory and circulatory systems network, leading to potential confusion due to high contrast images.
Our approach was to implement a pre-processing function that handles original images and annotations, isolating the lungs from the rest of the body using Hounsfield Units (HU) using a 3D U-NET segmentation algorithm and .postprocessing This detection allowed us to automatically reject artifacts detected outside the lungs.
This step was followed by a nodule detection model, based on the « Retina U-Net 3D » architecture https://www.nature.com/articles/s41591-019-0447-x , that accepted the preprocessed image input and predict the detected nodules by generating the coordinates of a rectangular parallelepipedal box, but also intensity attributes and segmentation results.
Finally, we used a classification model (SVM support vector machine after feature extraction), to classify the patient as pathological having at least one nodule greater than 100 mm3 or normal.
Thanks to IBM team we used 3 IBM Power AC922 servers with Nvidia V100 32 Go graphic cards (GPU – Graphics Processing Unit with respectively 4, 4 et 6, for a total of 14 GPUs)
https://www.ibm.com/us-en/marketplace/power-systems-ac922 .
That processing power together with the capacity to handle large models allowed to process successfully the test images in the required time with the best result of the ten teams in the challenge.
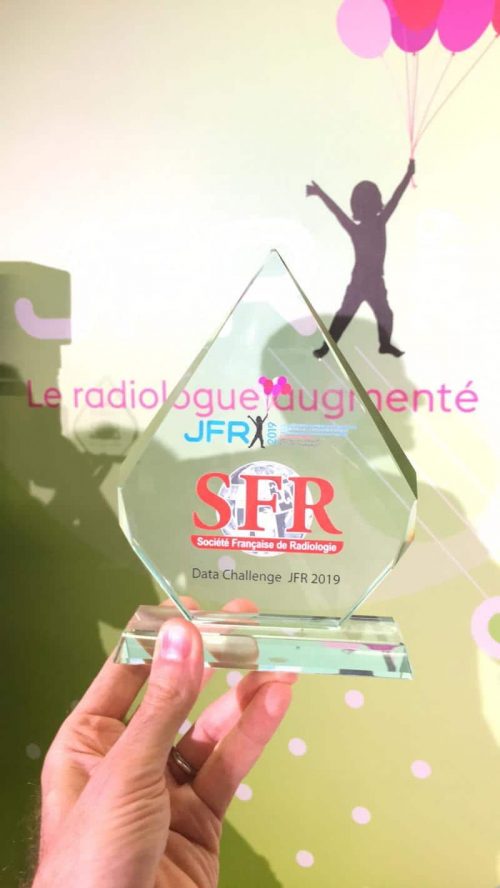
That processing power together with the capacity to handle large models allowed to process successfully the test images in the required time with the best result of the ten teams in the challenge.
The method was publish into the journal “Diagnostic and Interventional Imaging” in 2020 nov (DOI: 10.1016/j.diii.2020.10.004)
https://www.sciencedirect.com/science/article/pii/S2211568420302497
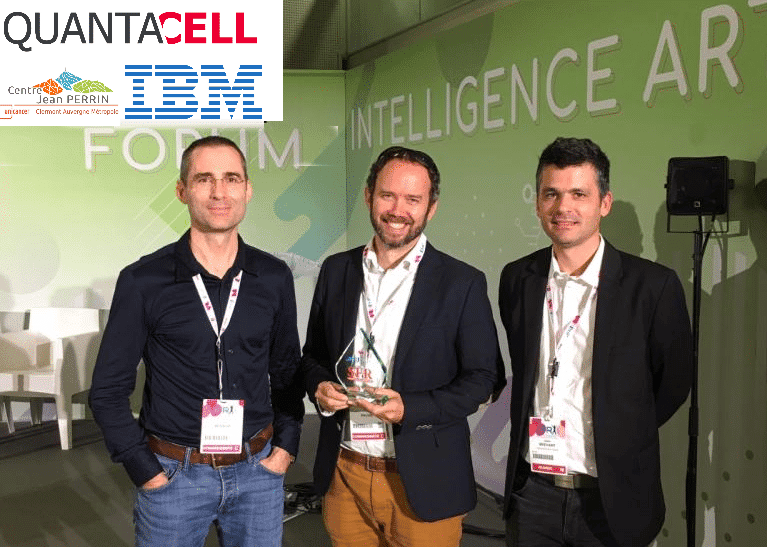
QuantaCell, Hôpital Saint Eloi, IRMB
80 av Augustin Fliche
34090 Montpellier, France
Contact
+33 (0) 9 83 33 81 90